- ListedAI Daily
- Posts
- From Molecular Models to Industry Disruption
From Molecular Models to Industry Disruption
MDGen and DeepSeek are reshaping science and AI alike innovation.
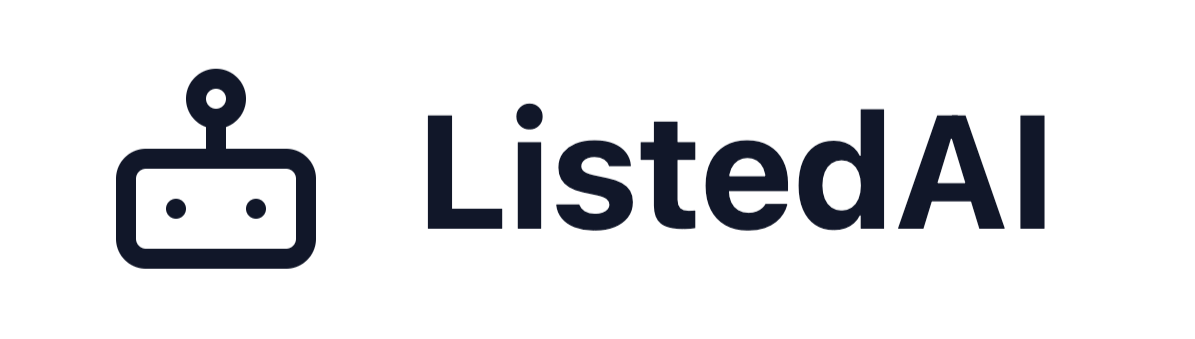
Wednesday Deep Dive
(Reading Time: 4 minutes)
The future of presentations, powered by AI
Gamma is a modern alternative to slides, powered by AI. Create beautiful and engaging presentations in minutes. Try it free today.
The Wednesday Deep Dive takes a detailed look at what's new in AI. Each week, we share in-depth insights on new tools, proven prompts, and significant developments - helping tech professionals work smarter and stay ahead.
This week, we’re uncovering two areas in AI research:
✨ MDGen: Generative AI Revolutionizes Molecular Simulations
🌐 DeepSeek: Redefining the Cost of AI
Let's dive in.
🌐 AI News
🔧 AI Brings Molecules to Life
Molecular dynamics just got a major boost from MIT’s latest generative AI model, MDGen, which simulates molecular motion with remarkable efficiency. This tool enables researchers to visualize and study molecular interactions—a process traditionally hindered by high computational costs and lengthy simulations.
MDGen uses generative AI to simulate the movements of molecules, turning static frames into dynamic “videos” that reveal molecular behaviors. Key features include:
Generative Modeling: MDGen predicts molecular trajectories between static frames, capturing interactions critical for drug design and material science.
Efficiency Gains: Simulates movements 10-100 times faster than traditional physics-based models, completing tasks in minutes instead of hours.
Advanced Features: MDGen can connect frames, upsample low frame-rate trajectories, and restore missing molecular structures through “inpainting.”
👍 Why It’s Exciting
MDGen represents a paradigm shift in scientific research:
Faster Drug Development: MDGen accelerates the discovery process for diseases like cancer or tuberculosis by simulating how molecules interact with drug prototypes.
Accessibility: Traditional molecular simulations require expensive supercomputers, but MDGen’s efficiency opens the door for smaller research teams.
Broader Applications: Beyond drug design, the tool offers potential in material science, protein engineering, and chemical analysis.
Additionally, MDGen’s ability to predict molecular movements with high accuracy can provide critical insights into dynamic processes that were previously too resource-intensive to model. By removing these barriers, researchers can focus on experimenting with new hypotheses rather than being constrained by computational limitations.
✨ Opportunities and Challenges
MDGen’s parallel generation approach marks a significant departure from traditional methods. Instead of relying on step-by-step simulations, it creates multiple frames simultaneously, making it more flexible and efficient for a variety of scientific applications.
Brand-New Applications: Chemists can visualize how drugs bind to molecular targets, offering better insights into effectiveness.
Scalability: While MDGen excels with small molecules, scaling it for complex protein dynamics remains a challenge. Researchers are working to expand its data and capabilities.
Accuracy vs. Scope: Current simulations are limited to toy systems, but the potential to generalize MDGen for broader use cases is on the horizon.
🚀 What’s Next?
MIT researchers aim to enhance MDGen’s scalability, enabling it to predict protein dynamics and simulate complex molecular systems. With plans to create larger datasets and refine its architecture, MDGen could redefine how science approaches molecular simulations. The potential impact on areas like precision medicine and green chemistry could be transformative, further pushing the boundaries of what’s possible in molecular research.
🌐 AI News
🌎 The Future of Cost-Efficient AI
China’s DeepSeek has sparked global conversations with two AI models, V3 and R1, that achieve groundbreaking performance at a fraction of the cost typically associated with advanced AI systems. DeepSeek’s achievements challenge industry norms and highlight the potential for efficiency-driven innovation.
DeepSeek is a Chinese AI startup that has upended the traditional AI development playbook by:
Cost Efficiency: Training R1 for just $5.6 million, compared to the $100+ million spent on models like OpenAI’s GPT-4.
Optimized Engineering: Leveraging techniques such as reinforcement learning and multi-head latent attention to maximize performance while minimizing computational demands.
Open-Source Influence: Using Meta’s Llama as a foundation and synthetic data for training, DeepSeek dramatically reduced the need for extensive compute resources.
🤔 Why It Matters
DeepSeek’s approach reshapes the competitive landscape of AI development:
Democratizing AI: Smaller startups can now compete with AI giants by focusing on smarter engineering rather than brute computational force.
Market Impact: DeepSeek’s cost-efficiency has already caused a $500 billion drop in Nvidia’s market cap, as investors reconsider the demand for high-end GPUs.
Innovation vs. Investment: The success of DeepSeek challenges the assumption that only massive budgets can yield cutting-edge AI, shifting focus to resource optimization.
DeepSeek’s reliance on synthetic data also raises interesting possibilities. By creating artificial datasets, the company sidestepped traditional limitations like data scarcity and intellectual property concerns. This approach could inspire other AI startups to experiment with similar strategies, further reducing entry barriers for innovation.
❌ Opportunities and Challenges
Beyond technical achievements, DeepSeek’s rapid success also serves as a reminder of the importance of agility in AI development. The company’s ability to replicate and enhance existing models within months underscores the value of streamlined operations and innovative problem-solving.
Industry Disruption: DeepSeek’s methods demonstrate that startups can achieve world-class results without the deep pockets of tech giants.
Transparency Concerns: Critics question the accuracy of DeepSeek’s claims and the implications of its reliance on open-source and synthetic data.
Geopolitical Dynamics: DeepSeek’s rise highlights China’s growing influence in AI, raising questions about global competition and technological dominance.
🚀 What’s Next?
DeepSeek’s success shows the importance of innovation in scaling AI efficiently. By focusing on smarter engineering and resource optimization, it has paved the way for a new generation of AI startups to challenge industry norms. As these techniques become more widely adopted, the AI landscape could shift from one dominated by a few large players to a more competitive and accessible ecosystem.
The next steps for DeepSeek involve scaling its infrastructure to handle greater demand, particularly as its models gain traction in the open-source community. The potential for broader collaboration could accelerate advancements, making sophisticated AI tools more widely available across industries.
Want to Unsubscribe from future emails? Just hit “unsubscribe” below.